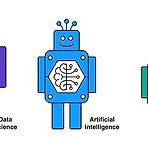
해당 게시글은 위 출처에서 번역하여 내용을 정리하였음을 밝힙니다. Data Science vs. Machine Learning Data science는 unstructured/raw data(비정형/원시 데이터)에서 정보를 추출하는 프로세스입니다. 이 작업을 수행하기 위해 여러 algorithms, machine learning technique, data analysis를 통합합니다. 그래서 개념으로 따지면 Data Science > Artificial Intelligence > Machine Learning 의 순으로 큰 개념이라 볼 수 있습니다. Definition of Data Science & Machine Learning Data science는 여러 분야를 통합한 개념으로 과학적인 방법, a..
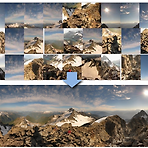
| Today's topic is Features extraction - Corners and blobs. | Key points feature extraction local feature Harris corner detection Motivation: Automatic panoramas The human visual system has a field of view of around 135 x 200 degrees, but a typical camera has a field of view of only 35 x 50 degrees. Panoramic image mosaicing works by taking lots of pictures from an ordinary camera, and stitching..
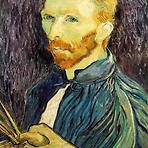
| Today's topic is Sampling & interpolation. | Key points Subsampling an image can cause aliasing. Better is to blur (“pre-filter”) to remote high frequencies then downsample If you repeatedly blur and downsample by 2x, you get a Gaussian pyramid Upsampling an image requires interpolation. This can be posed as convolution with a “reconstruction kernel” Image scaling This image is too big to fit ..
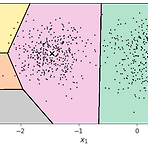
비지도 학습(unsupervised learning)에 대한 파트입니다. 챕터 8에서 살펴본 차원 축소(dimension reduction)도 가장 널리 사용되는 unsupervised learning에 해당됩니다. 이번 장에서는 몇 가지 학습과 알고리즘을 살펴봅니다. 저는 책의 내용이 너무 방대하고 이 이론을 뒷받침하기엔 설명이 부족하다고 판단하여 주요 개념만 다루려고 합니다. 저는 Clustering, K-means, DBSCAN, GMM을 간단히 요약 정리하였습니다. 1. Overview Unsupervised learing의 문제 클러스터링(clustering) 차원 압축(dimensionality reduction) 이상 감지(anomaly detection) Unsupervised learin..
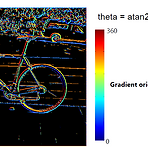
Edge detection Convert a 2D image into a set of curves Extracts salient features of the scene More compact than pixels Origin of edges Edges are caused by a variety of factors Images as functions Edges look like steep cliffs Characterizing edges An edge is a place of rapid change in the image intensity function Image derivatives How can we differentiate a digital image $F[x,y]$ Option 1: reconst..
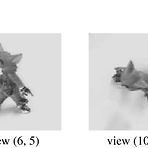
1. What is an image? A grid (matrix) of intensity values (common to use one byte per value: 0 = black, 255 = white) Can think of a (grayscale) image as a function $f$ from $R^2$ to $R$ $f(x,y)$ gives the intensity at position (x,y) A digital image is a discrete (sampled, quantized) version of this function Image transformations As with any function, we can apply perators to an image Today we'll ..
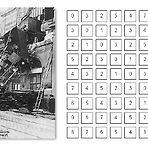
What is computer vision? Why study computer vision? Course overview Images & Image filtering [time permitting] 1. What is computer vision? Goal of computer vision: percieve the story behind the picture Compute properties of the world 3D shape Names of people or objects What happened? Can computers match human perception? Yes and No (maninly no) computers can be better at 'easy' things humans are..
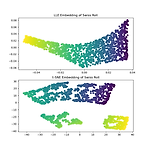
핸즈온머신러닝의 챕터 8을 공부하고 정리합니다. 참고한 글은 아래 Reference로 남겨두었습니다. 이번 챕터 8의 주제는 Dimension Reduction입니다. 책의 목차는 아래와 같습니다. 8.0 개요 8.1 차원의 저주 8.2 차원 축소를 위한 접근 방법 8.3 PCA 8.4 커널 PCA 8.5 LLE 8.6 다른 차원 축소 기법 책 내용을 그대로 정리하진 않을 것 같고, 중요하다고 생각하는 키워드와 개념만 뽑아서 정리해보도록 하겠습니다. | Keyword | Curse of dimensionality, Projection, Manifold, PCA, Kernel PCA, LLE Curse of dimensionality 차원이란 말은 각 sample을 정의하는 정보들로 생각할 수 있습니다. ..
- Total
- Today
- Yesterday
- NLP
- 서버구글드라이브연동
- 파이썬 클래스 계층 구조
- support set
- Prompt
- 파이썬
- vscode 자동 저장
- stylegan
- 프롬프트
- 데이터셋다운로드
- 퓨샷러닝
- clip
- docker
- 구글드라이브서버다운
- style transfer
- 서버에다운
- CNN
- few-shot learning
- prompt learning
- python
- Unsupervised learning
- 파이썬 클래스 다형성
- 파이썬 딕셔너리
- 구글드라이브다운
- 구글드라이브연동
- 도커
- 도커 컨테이너
- 구글드라이브서버연동
- cs231n
- 딥러닝
일 | 월 | 화 | 수 | 목 | 금 | 토 |
---|---|---|---|---|---|---|
1 | 2 | 3 | 4 | |||
5 | 6 | 7 | 8 | 9 | 10 | 11 |
12 | 13 | 14 | 15 | 16 | 17 | 18 |
19 | 20 | 21 | 22 | 23 | 24 | 25 |
26 | 27 | 28 | 29 | 30 | 31 |