티스토리 뷰
[논문 리뷰] A Style-Based Generator Architecture for Generative Adversarial Networks
미남잉 2023. 5. 18. 22:49- Paper: https://arxiv.org/abs/1812.04948
- Video: https://youtu.be/kSLJriaOumA
- Code: https://github.com/NVlabs/stylegan
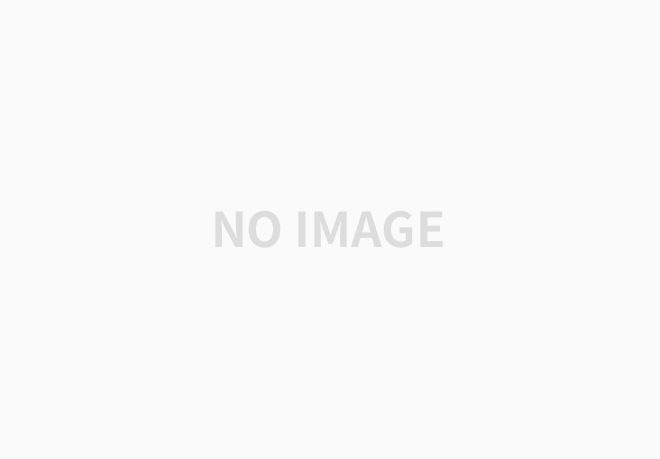
StyleGAN의 output images이다.
"A Style-Based Generator Architecture for Generative Adversarial Networks"은 Ian J. Goodfellow 등의 저자들이 2019년에 발표한 논문이다. 이 논문은 기존의 생성적 적대 신경망(GANs)의 생성자 아키텍처를 개선하여 고해상도 이미지 생성의 질과 다양성을 향상시키는 새로운 접근 방식을 제안한다.
이 논문에서 제안하는 아키텍처는 "style-based generator"로 알려져 있는데, 해당 아키텍처는 기존의 GAN 생성자 아키텍처에 스타일 정보를 조절하는 메커니즘을 도입함으로써 이미지 생성에 큰 장점을 제공한다.
이를 위해 먼저, 랜덤 벡터 대신에 잠재 공간의 스타일 정보를 통해 이미지를 생성하는 스타일 잠재 공간을 도입한다. 스타일 잠재 공간은 스타일 벡터와 세부 사항 벡터로 구성된다. 스타일 벡터는 이미지의 전반적인 스타일에 영향을 주는 요소를 캡처하고, 세부 사항 벡터는 이미지의 미세한 픽셀 레벨 세부 정보에 영향을 준다.
이러한 스타일과 세부 사항 벡터는 네트워크의 다양한 계층에서 조절되어 이미지 생성에 큰 유연성을 제공한다. 논문에서는 또한 스타일 잠재 공간의 보간을 통해 이미지 간의 부드러운 전환을 가능하게 하는 스타일 전이의 개념을 제안한다. 이를 통해 두 개의 다른 이미지의 스타일과 세부 사항을 조합하여 새로운 이미지를 생성할 수 있다.
또한, 스타일 잠재 공간에서 스타일 벡터의 특정 요소를 조작함으로써 이미지의 스타일을 조절하는 기능을 제공한다. 제안된 스타일 기반 생성자 아키텍처는 고해상도 이미지 생성에 많은 발전을 이끌었으며, 실제로 이미지 생성 작업에서 많은 성과를 보였다. 또한, 스타일 전이 및 스타일 조절과 같은 응용 분야에서도 많은 관심을 받았다.
Generative adversarial networks learn to generate entirely new images that mimic the appearance of real photos. However, they offer very limited control over the generated images. We came up with a new generator that automatically learns to separate different aspects of the images without any human supervision. After training, we can combine these aspects in any way we like. All images in this video were produced by our generator, they are not photographs of real people.
동영상 초입에 삽입된 자막입니다.
가볍게 내용을 살펴보면, 두 타입의 Source image가 있고 A, B가 합쳐진 결과가 나옵니다. A를 바탕으로 B의 스타일 전부를 흡수에서 결과가 나옵니다. content image를 받은 style transfer와 비슷해 보입니다. A의 종류로는 gender, age, hair length, glasses, pose 등이 있습니다.
따라서 이런 설명이 덧붙여집니다.
Our generator thinks of an imagesas a collection of "styles", where each style controls the effects at a particular scale.
- Coarse styles → pose, hair, face shape
- Middle styles → facial features, eyes
- Fine styles → color scheme
초반과 중간, 마지막으로 갈수록 더 세부적인 스타일을 학습하게 됩니다.
Additionally, our generator automatically separates inconsequential variation from high-level attributes (pose, identity, etc.)
- Coarse noise → large-scale curling of hair
- Fine noise → finer details, texture
- No noise→ featureless "painterly" look
따라서 같은 이미지도 noise input에 따른 다른 관계를 가진다는 것을 확인할 수 있었습니다.
We can choose the strength at which each style is applied, with respect to an "average face"
- High strength → maximal variation, some broken images
- Low strength → reduced variation, no broken images
- Negative strength → "anti-face"
By selecting the strength appropriately, we can get good images out every time (with slightly reduced variation)
간단히 살펴보았음
'Review > Paper' 카테고리의 다른 글
- Total
- Today
- Yesterday
- support set
- NLP
- 프롬프트
- 파이썬
- 리눅스 나노
- 도커 작업
- style transfer
- 도커 컨테이너
- 파이썬 클래스 다형성
- linux nano
- CNN
- 딥러닝
- 리눅스 nano
- 파이썬 클래스 계층 구조
- 리눅스
- 구글드라이브연동
- Prompt
- 서버구글드라이브연동
- 리눅스 나노 사용
- Unsupervised learning
- clip
- 도커
- python
- stylegan
- cs231n
- 퓨샷러닝
- few-shot learning
- docker
- 파이썬 딕셔너리
- prompt learning
일 | 월 | 화 | 수 | 목 | 금 | 토 |
---|---|---|---|---|---|---|
1 | 2 | 3 | 4 | 5 | ||
6 | 7 | 8 | 9 | 10 | 11 | 12 |
13 | 14 | 15 | 16 | 17 | 18 | 19 |
20 | 21 | 22 | 23 | 24 | 25 | 26 |
27 | 28 | 29 | 30 |